01 Jun 2023
Join us in Lisbon for the first annual MDAnalysis user group meeting (UGM) to hear fantastic talks from experts in the molecular dynamics simulations space. We are proud to introduce our first keynote speaker, Prof. Giovanni M. Pavan of the @GMPavanLab. Please register and submit your abstract soon to secure your place (Abstract Deadline: July 7, 2023) - you won’t want to miss out!
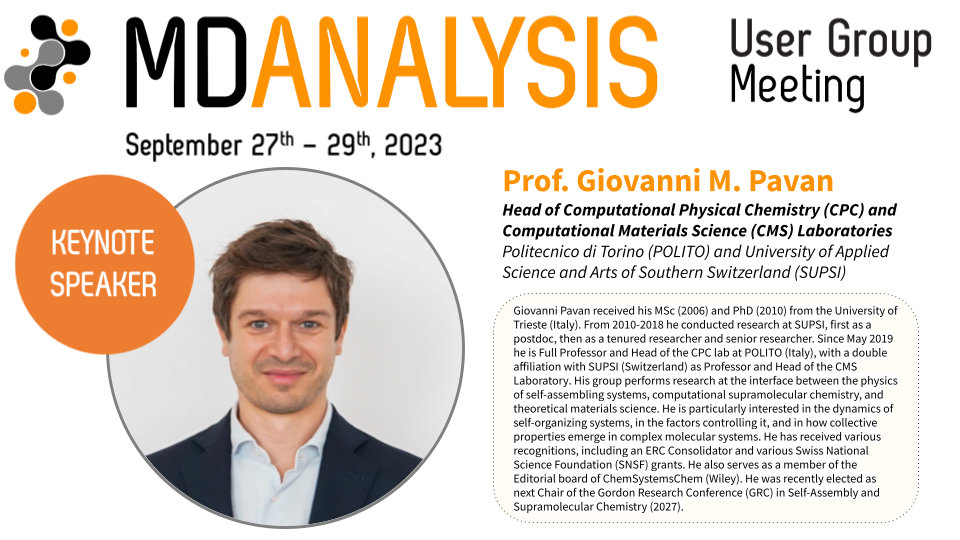
25 May 2023
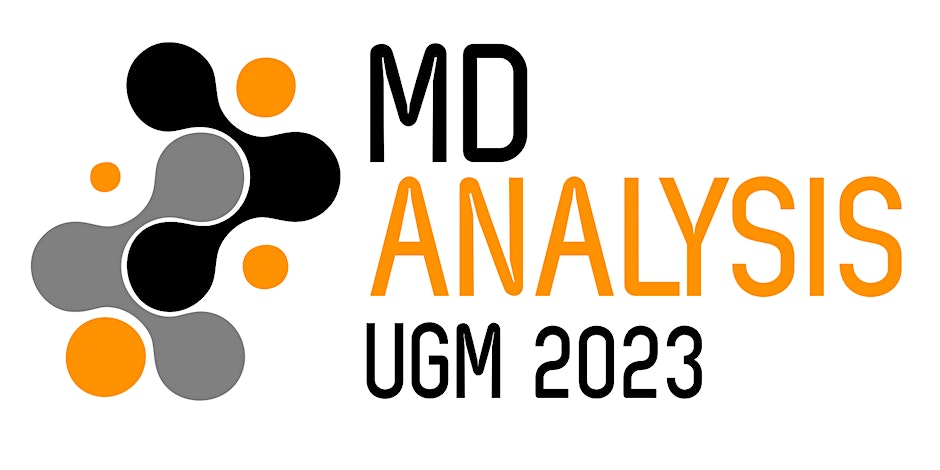
We are excited to announce that registration is now open for MDAnalysis’s inaugural user group meeting (UGM)! The meeting will be held in-person on September 27-29, 2023 in the Faculty of Sciences of the University of Lisbon Auditorium in Building C6 (Edificio C6, Faculdade de Ciências da Universidade de Lisboa, Campo Grande, 1749-016 Lisboa).
The UGM will bring together users of the MDAnalysis package from different communities. Our goal is to foster an opportunity to connect for interdisciplinary researchers and developers across molecular simulations, soft matter, biophysics and more.
MDAnalysis strives to be a diverse and welcoming community for all. While the event will be free to attend, a limited number of bursaries are available to enable attendance for those facing financial barriers. If you would like to apply, please follow the prompts on the registration form to submit your bursary application by June 23, 2023.
Follow our official event page on our website for the most up-to-date information about the UGM. If you have any questions or special requests, you may contact [email protected]. We look forward to seeing you in Lisbon!
18 May 2023
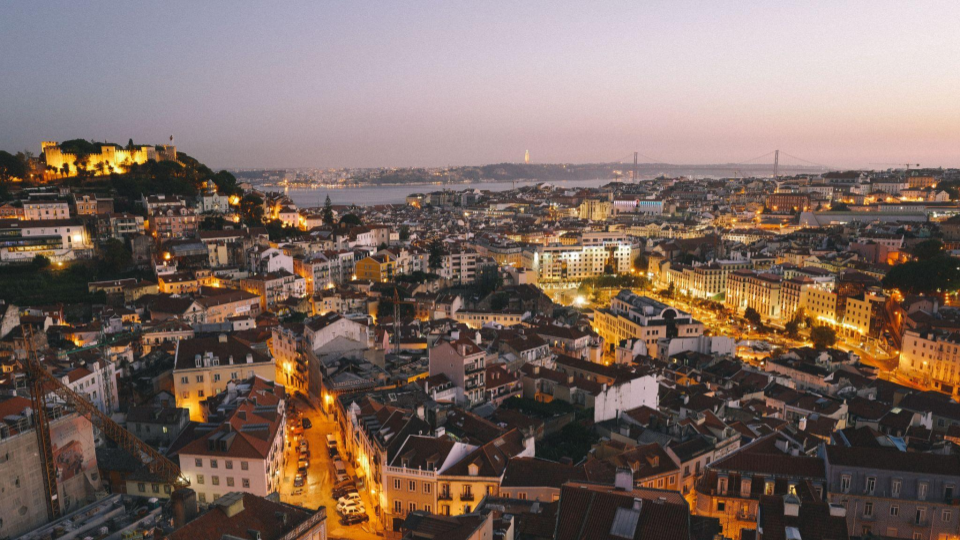
Photo by Andreas Brücker on Unsplash
We are thrilled to announce the first ever MDAnalysis User Group Meeting (UGM)! The MDAnalysis UGM 2023 will take place September 27-29 (Wednesday-Friday) at the University of Lisbon (Portugal). To foster a connection opportunity for interdisciplinary researchers and developers across biomolecular simulations, soft matter and biophysics, this year’s event will be held in-person.
What to Expect
Whether you are a new MDAnalysis contributor, an MDAnalysis core developer, or a researcher using (or interested in using) MDAnalysis for your work in academia or industry, this UGM is for you. You can expect:
- Keynote talks from experts in the molecular dynamics simulations space
- Talks related to developing and using MDAnalysis for diverse scientific applications
- Tutorials diving into new features
- Direct access to the MDAnalysis core developer team to help shape future development of MDAnalysis
- Networking opportunities to foster new collaborations
- Hackathon and other social outings
Additional details of the schedule and call for proposals will be communicated soon. In the meantime, save the date and keep an eye out for additional announcements on our blog and UGM website page, as well as on our Twitter and LinkedIn accounts.