01 Feb 2024
We are now accepting abstracts for the 2024 MDAnalysis UGM (User Group Meeting), taking place August 21-23, 2024, in London, UK at King’s College London. Abstracts are welcomed from all areas relevant to MDAnalysis’s work, from scientific applications (e.g., biomolecular simulations, soft matter and materials science, drug discovery and more) to the use and development of open source software and tools for molecular simulations data analysis. When submitting your abstract, you may indicate whether you prefer to give a 15 minute talk, 5 minute lighting talk, or poster presentation.
MDAnalysis strives to be a diverse and welcoming community for all. While the event will be free to attend, a limited number of travel bursaries are available to enable those facing financial barriers to attend and present their work. If you would like to apply, please follow the prompts on the abstract submission form.
The deadline for submitting your abstract and/or bursary application is March 15, 2024. Submissions will be reviewed by the end of March 2024; we will contact applicants shortly after.
Follow our official event page on our website for the most up-to-date information about the UGM. If you have any questions or special requests, you may contact [email protected].
18 Jan 2024
Contributor: Xu Hong Chen
Mentors: Hugo MacDermott-Opeskin (@hmacdope), Orion Cohen (@orionarcher)
Organization: MDAnalysis
Transport property analyses are among the most requested features in the MDAnalysis project. Biomolecular researchers and chemical engineers routinely use these measurable values describing the transfer of mass, momentum, heat, and charge in their regular work., My Google Summer of Code (GSoC) project aimed to add methods for calculating self-diffusivity and viscosity, the two most common transport properties in the literature, to MDAnalysis with a focus on user-friendliness and test-driven development. Initially, I proposed to:
- Implement a class to calculate self-diffusivity via the Green-Kubo method
- Write a utility function to calculate shear viscosity via the Einstein method
- Create a utility function to calculate shear viscosity via the Green-Kubo method
- Test and document the new features to ensure they are reliable and easy to use
After some discussion that began with a suggestion from @jaclark5 to try the Helfand method for viscosity to better integrate the feature wtih MDAnalysis, we shifted our priorities away from the utility functions to focus on a new Einstein-Helfand viscosity class. That extra research and trial and error were well worth the result - better software with a more simple and robust API.
My Summer in Python and NumPy Arrays
My summer culminated in the creation of Transport Analysis, a standalone MDAKit Python package to compute and analyze transport properties powered by MDAnalysis.
Transport Analysis GitHub: https://github.com/MDAnalysis/transport-analysis
Building a FAIR-compliant Python Package from the MDAKit Cookiecutter Template
Generating my package from the MDAKit cookiecutter template streamlined package creation, ensuring that Transport Analysis was set up through a reliable, stringently reviewed process. The included CI/CD pipeline needed some adjusting on GitHub, but it facilitated writing maintainable software from the very beginning. Pull request (PR) #3 added the new code formatter Black to the CI, while PR #22 flattened the package’s structure to simplify its organization of modules.
Velocity Autocorrelation Functions (VACFs) and Green-Kubo Self-Diffusivity
PR #1 built two implementations of the VACF calculation: a simple “windowed” algorithm and a fast FFT-based algorithm leveraging tidynamics
. All the computations involved are vectorized, but the FFT-based algorithm speeds the calculations up even further. I designed a simple test system from a unit velocity trajectory and began with some very basic calculations on pen and paper, then gradually wrote more and more tests until my code was tested with the same rigour as the MDAnalysis MSD module on top of reaching 100% code coverage.
PR #23 introduced a plotting method for VACFs via Matplotlib
’s object-oriented API, saving users from having to write their own plotting code. PR #24 then makes it easy to calculate the Green-Kubo self-diffusivity with the methods self_diffusivity_gk
and self_diffusivity_gk_odd
. It also added a new plotting method for the running integral.
Einstein-Helfand Viscosity
PR #25 was challenging because it was not part of the initial plan and the methodology and practices were less prominent in the literature. The calculation itself was also more difficult to work with because it had a lot more terms than self-diffusivity, but I enjoyed the process of learning and developing this class. Like the VACF implementation, it takes full advantage of NumPy arrays and the MDAnalysis AnalysisBase API.
PR #29, which adds plotting functionalities for the Einstein-Helfand viscosity class, is essentially complete but has yet to be merged because we are still considering whether it would be better to leave the plot in standard MDAnalysis units or convert them to SI units. While standard MDAnalysis units are friendly for VACFs and self-diffusivity, that is not the case for viscosity due to the number of terms involved in the calculation. It is the only unmerged PR in the project at the time of writing.
Because we prioritized the new, more user-friendly Einstein-Helfand viscosity class, which turned out to be more time consuming due to the research and complex implementation, I did not get to write the Green-Kubo viscosity utility function within the GSoC timeline. Nonetheless, I am happy to have adapted my original plan to our community discussions and delivered a more useful project. I plan to implement the utility function after GSoC, though I suspect most users will much prefer the Einstein-Helfand viscosity class. Ultimately, I achieved my goals for this summer and I am excited to see Transport Analysis grow as a community resource.
Reproduction of the Literature
To go beyond testing against simple “toy” systems and test data, I began a reproduction of established transport property calculations in the literature to validate my implementations. I successfully calculated a self-diffusivity of $2.47 \times 10^-9$ $m^2 / s$ using my Green-Kubo self-diffusivity implementation from a $20$ $ps$ simulation of the SPC/E water model at $298$ $K$, which agrees well with the results in the literature., A full writeup of this reproduction can be found in my last blog post, Reproduction and Beyond GSoC (GSoC ‘23, 4). Some of the key plots are provided below.
VACF Plot
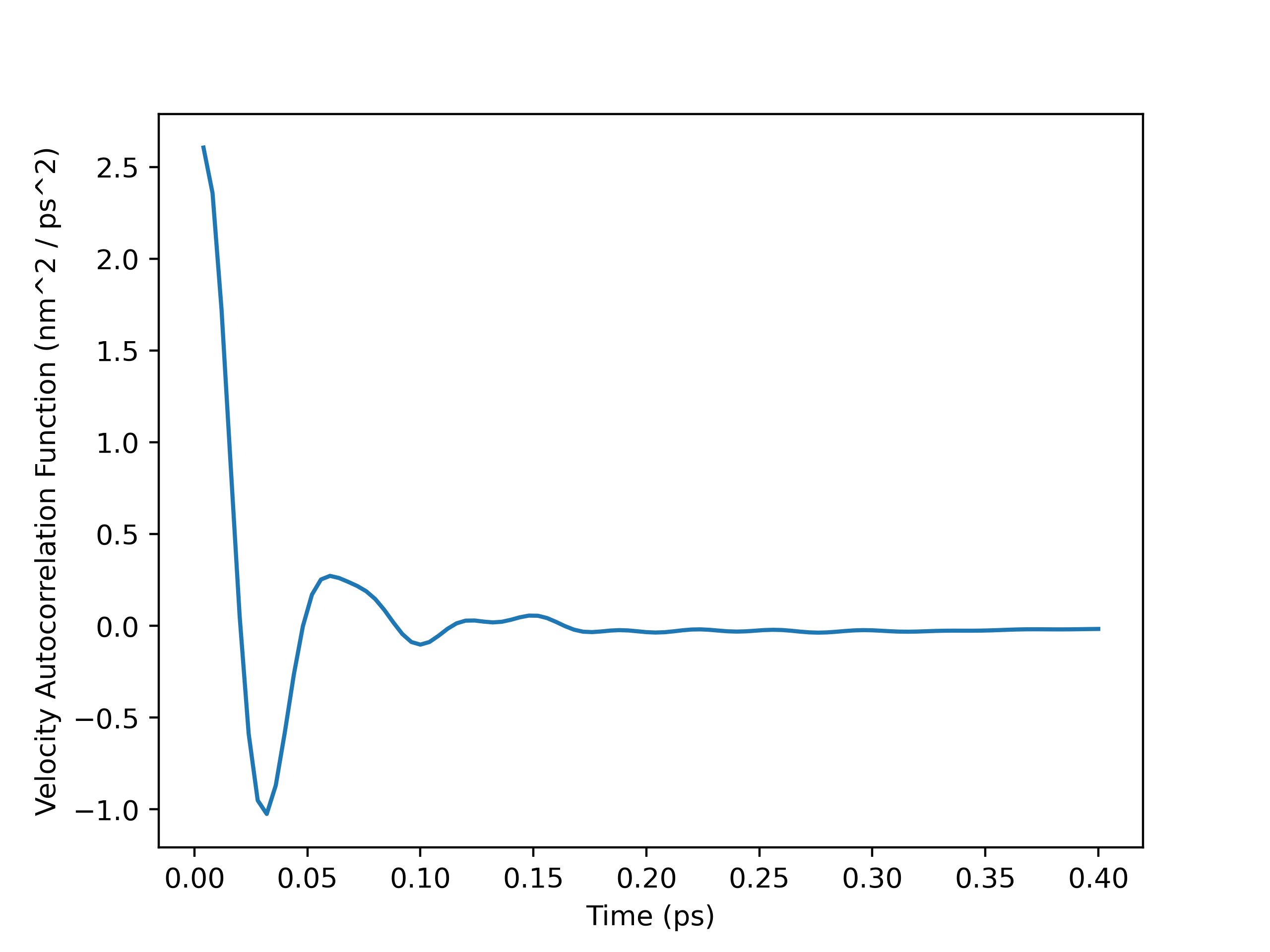
Running Integral Plot
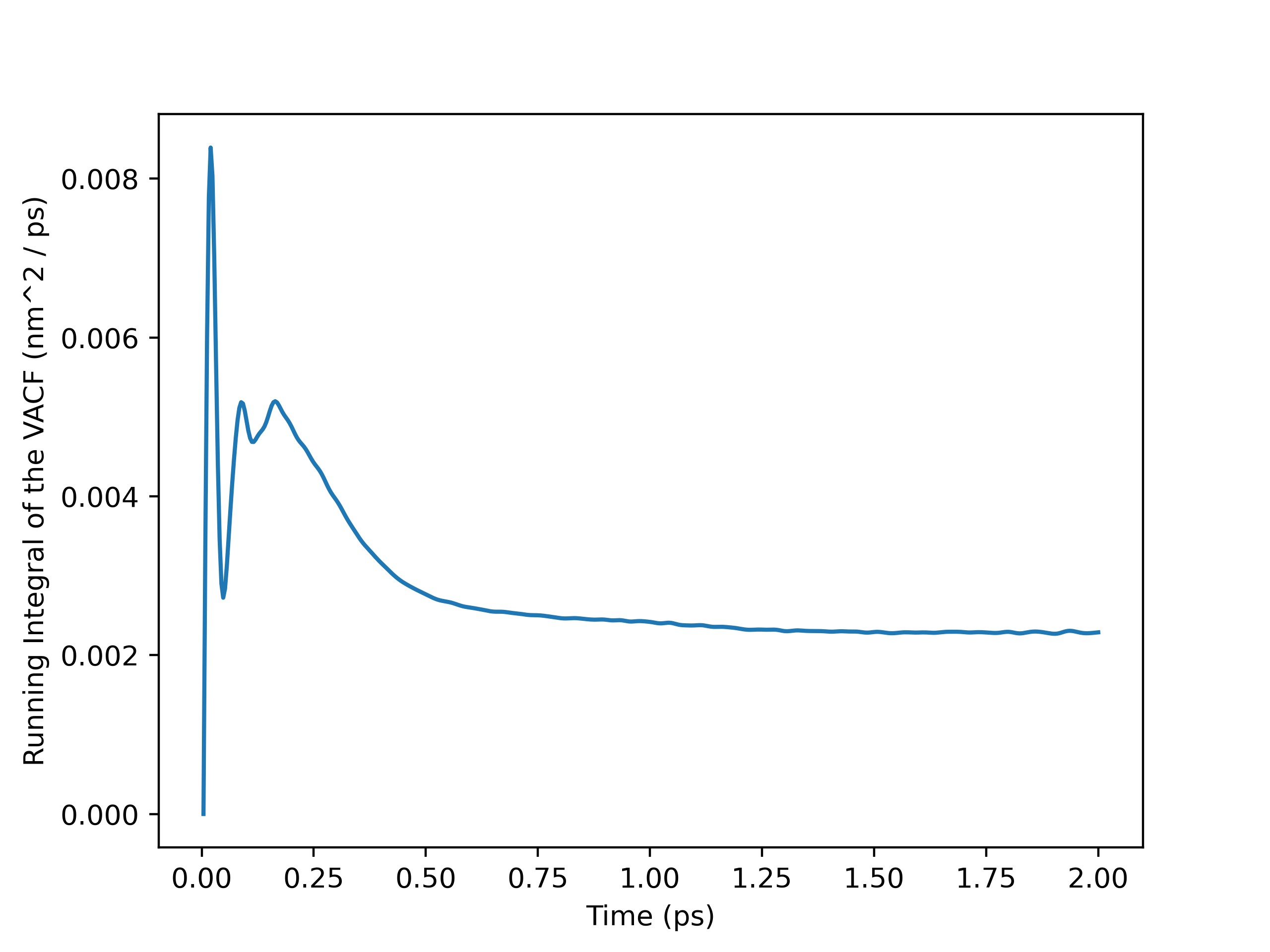
Using Transport Analysis
Installation
Transport Analysis is released and available on PyPi; the latest version of the package can be installed with pip
:
pip install transport-analysis
A Quick VACF Example
This example calculates a VACF from a very small set of AMBER test data with velocities. In Python, execute:
# imports
import MDAnalysis as mda
from transport_analysis.velocityautocorr import VelocityAutocorr
# test data for this example
from MDAnalysis.tests.datafiles import PRM_NCBOX, TRJ_NCBOX
We will calculate the VACF of an atom group of all water atoms in residues 1-5. To select these atoms:
u = mda.Universe(PRM_NCBOX, TRJ_NCBOX)
ag = u.select_atoms("resname WAT and resid 1-5")
We can run the calculation using any variable of choice such as wat_vacf
and access our results using wat_vacf.results.timeseries
:
wat_vacf = VelocityAutocorr(ag).run()
wat_vacf.results.timeseries
# results from wat_vacf.results.timeseries
array([275.62075467, -18.42008255, -23.94383428, 41.41415381,
-2.3164344 , -35.66393559, -22.66874897, -3.97575003,
6.57888933, -5.29065096])
Notice that this example data is insufficient to provide a well-defined VACF. When working with real data, ensure that the velocities are written frequently enough to obtain a VACF suitable for your needs.
A growing collection of Jupyter Notebook tutorials/examples can be found in the tutorials directory in the repository.
Lessons Learned
Here are a few highlights from my series of blog posts:
- Always check if the dependencies used are up-to-date and/or the expected version
- Look online to see if there are any well-maintained, trustworthy packages as reference for the feature to be implemented (e.g. the MSD module)
- Ask a lot of questions - many of the mentors who were not assigned to my project helped
- Following the GitHub discussions that more experienced developers have can be a fantastic learning opportunity
- Having a call with someone more experienced, like Hugo, when starting something completely new can really streamline the learning process
- Approaching your software with a real use case from the perspective of a user is a wonderful way to find new areas for improvement
The Future
The beauty of open source is the potential for continued improvement and engagement with the community. I will continue working on Transport Analysis after GSoC, and both my mentors intend to make their own contributions to the package. We are always open to new ideas, GitHub issues, bug reports, and contributions. The following is simply a list of a few of the many possibilities for improving this community resource:
- Implement a utility function to calculate viscosity via the Green-Kubo method (planned)
- Reproduce literature results using the implemented Einstein-Helfand viscosity class (will continue looking into in consultation with MDAnalysis community)
- Implement additional transport property analyses (ionic and thermal conductivity, etc) and tools (utility functions, plotting functions, etc)
- Continue adding example Jupyter Notebooks
- Continue improving the documentation
Acknowledgements
I would like to thank MDAnalysis for supporting me from my first PR to my completion of GSoC and beyond. It has been such a wonderful experience discussing software and science on GitHub, Discord, and the mailing lists. I am very grateful for the continued support of my mentors, Hugo MacDermott-Opeskin (@hmacdope) and Orion Cohen (@orionarcher), who have taken the time to have many live calls with me, carefully review my code and the science of my work, and share a wealth of lessons and resources that I will continue learning from well after GSoC.
I would like to extend further thanks to Oliver Beckstein (@orbeckst) for his support throughout my involvement with MDAnalysis and his insights on computational biophysics and programming. I also really appreciated Lily Wang (@lilyminium) and Irfan Alibay’s (@IAlibay) help with setting up the package and the CI. I enjoyed talking about code formatting with Rocco Meli (@RMeli) and I am grateful for Richard Gowers’ (@richardjgowers) reviews of my first analysis class. Finally, I would like to thank Google for offering this program and supporting open-source software.
If any readers are interested in scientific software, molecular simulations, or computational research, I would highly encourage looking into the MDAnalysis community. The developers’ dedication and ability shows in the library’s impressive performance compared to similar software and its unmatched interoperability and support for a wide range of analyses.
– @xhgchen
References
18 Jan 2024
As you might know from my previous posts, during the summer of 2023 I’ve been working on MDAnalysis during Google Summer of Code. Here I’ll summarize what I’ve done, how others can use it, and what changes will follow that in the MDAnalysis codebase in the near future.
Goals of the project
One sentence: introduce parallel execution of analysis runs in MDAnalysis library. Somewhat good introduction I also gave here when writing a proposal for the project.
In more technical details, MDAnalysis library (as of v2.6.0) contains around 30 different subclasses that can perform various molecular trajectory analysis tasks, like calculating RMSD, RMSF, various contacts, density analysis, and more advanced tasks. 24 of these subclasses are children of AnalysisBase
class. This base class is written in a way that allows subclass authors care about implementing only 3 methods:
-
_prepare()
– how to initialize attributes for the analysis
-
_single_frame()
– how to do analysis of a single molecular trajectory frame
-
_conclude()
– how to transform intermediate results into final ones
With only these 3 methods implemented, by inheritance subclasses get run()
method that will take care of reading the trajectory, storing the results, logging, etc.
The main goal was to re-write AnalysisBase.run()
so that it can run in parallel on multiple processes, but make these changes invisible from the subclasses, i.e. not re-write any of their code.
What I did
Altogether, the AnalysisBase.run()
has changed by addition of the following methods:
-
_setup_computation_groups()
: split frames into multiple parts for separate analysis
-
_compute()
: run _single_frame
on a list of frames, but without running _conclude
-
_get_aggregator()
: get an object to aggregate the run results with, making them compatible with subsequent _conclude
- class property
available_backends()
: get list of str
values that describe available backends for a given subclass
I’ve also added ParallelExecutor
and ResultsGroup
classes that abstract away parallel execution and results aggregation, respectively. And finally, I added multiprocessing
and dask
backends that reportedly speed up the analysis!
The current state
Currently, changes to the AnalysisBase
are almost finalized. One thing that holds it back is some CI/CD issues causing tests to timeout, but all AnalysisBase
-related tests run both locally and on CI/CD system.
What’s left to do
An optional thing suggested within my proposal was to actually add parallelization to the subclasses, and update tests accordingly. It turned out to be a tedious task, but finally the mechanism is finalized and described in MDAnalysisTests/analysis/conftest.py
, generating appropriate fixtures for testing subclasses with all supported (for the subclass) and installed (in the environment) backends.
Since it is a big change for the library, essentially changing the API for the “analysis” part of the MDAnalysis, it requires a lot of time to merge into the develop branch, and wasn’t merged within the GSoC timeframe. But the feature is expected by version 3.0 in the core library 🚀
What code got merged (or not) upstream
The main changes are summarized in the main pull-request of the project. They mostly involve changes to package/analysis/base.py
, as well as installation and CI/CD configuration files. Also, there are example changes in package/analysis/rms.py
introducing parallelization into RMSD and RMSF subclasses, showcasing the changes to be made in order to add parallelization to a certain class.
How can you use it?
Let’s imagine we’ve just learned that MDAnalysis now supports parallelization, and want to calculate RMSD of our large trajectory faster using multiple cores on our machine. Imagine we have a 16-core CPU Linux workstation with an SSD drive and a 1 us trajectory of lysozyme in water. Like this:
import MDAnalysis as mda
from MDAnalysis.analysis import rms
prefix = "./large_data/"
traj, top = f"{prefix}/md_0_1.xtc", f"{prefix}/md_0_1.gro"
u = mda.Universe(top, traj)
First we want to get a reference structure by taking the average of all frames. Like this:
from MDAnalysis.analysis.align import AverageStructure
avg = AverageStructure(mobile=u).run(backend='multiprocessing', n_workers=16)
but we get this:
ValueError Traceback (most recent call last)
Cell In[11], line 2
1 from MDAnalysis.analysis.align import AverageStructure
2 avg = AverageStructure(mobile=u).run(backend='multiprocessing', n_workers=16)
...
ValueError: backend=multiprocessing is not in self.available_backends=('local',) for class AverageStructure
which basically says we can use only backend='local'
for the AverageStructure
. Ok, let’s do that, but with a large step to save time:
avg = AverageStructure(mobile=u).run(step=100)
ref = avg.results.universe
and start our analysis run – RMSD for multiple selections to later compare them between each other:
groupselections = ("protein", "backbone", "name CA")
R = rms.RMSD(
u, # universe to align
ref, # reference universe or atomgroup
groupselections=groupselections,
select="backbone", # group to superimpose and calculate RMSD
)
If we start it with R.run()
, we won’t even know when the run would finish. Luckily, we can add some verbosity with R.run(verbose=True)
and see a nice tqdm
progressbar that shows us that ETA of the whole analysis is around 4 minutes:
>>> R.run(verbose=True)
5%|▌ | 5062/100001 [00:13<04:15, 371.20it/s]
Let’s try to speed it up now. Which backends do we have available?
>>> rms.RMSD.available_backends
('local', 'multiprocessing', 'dask')
Let’s try a built-in multiprocessing
first:
>>> R.run(backend='multiprocessing', n_workers=4)
# CPU times: user 153 ms, sys: 74.2 ms, total: 227 ms
# Wall time: 1min 14s
OK, this is roughly 4 times faster! Amazing, roughly as we expected.
Spoiler though – if we do it with 16 workers, we’ll see the total time around 40 seconds, so improvement saturates at some point.
But, we’ve lost something valuable when switching to multiprocessing
– we don’t have a decent progressbar anymore. Luckily, we can use an amazing dask
dashboard that allows us to monitor all tasks given to a particular dask.distributed
cluster!
Let’s set up a cluster first:
from dask.distributed import Client, LocalCluster
cluster = LocalCluster(n_workers=8,
threads_per_worker=1,
memory_limit='30Gb')
client = Client(cluster)
and open the dashboard in our browser:
>>> cluster.dashboard_link
'http://127.0.0.1:8787/status'
Now, we’re ready to pass the pre-configured client
as an argument to our R.run()
:
Unfortunately, we won’t see much progress – we can see that all tasks got spawned, but their status will change only upon completion, and we won’t get any intermediate progress report.
But luckily, there is a way to control that: in R.run()
function, you can split the workload into an arbitrary number of parts with n_parts = ...
, and upon completion of each of them dask
would report that. Let’s do this:
R.run(client=client, n_parts=96)
Now we’ll see intermediate progress as well as soon as each part gets completed, which is super helpful when trying to estimate the completion time.
Conclusion
We’ve now gone through the essence of the MDAnalysis parallelization project, and learned how to use it in your analysis either by simply adding backend='multiprocessing', n_workers=...
, or setting up your own dask
cluster and submitting your jobs there.
Hopefully, this project will grow further and include all existing subclasses, as well as improving the speed (which, as we saw, saturates) and memory efficiency.
If you want to contribute to the project, stay tuned for the new issues on MDAnalysis github – there definitely will be some parallelization-related things for the future described there!
– @marinegor